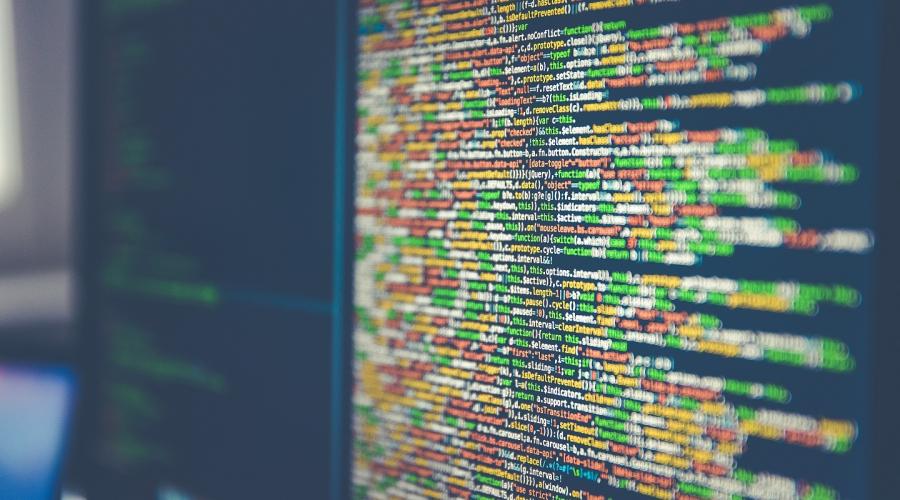
Predicting Early Deliveries: Using Machine Learning to Find Pregnancies at Risk
Despite preterm birth being the leading cause of infant mortality worldwide, clinicians have difficulty determining which pregnancies are at risk and would benefit from additional support. A crowd-sourced effort using machine learning looks to change that.
An international team led by Marina Sirota, PhD, an associate professor of pediatrics with the Bakar Computational Health Sciences Institute at UC San Francisco, organized a global challenge for researchers to develop machine learning models that can predict which pregnancies are likely to have preterm delivery based on microbiome data.
Sirota says “the ability to predict pregnancies at risk of preterm birth is a crucial first step in developing and implementing prevention strategies. There are some known factors associated with preterm birth, but clinical tools are needed to assess the risk for individuals, early and reliably.”
The top performing predictive models had up to 87% accuracy when predicting early preterm birth (births before 32 weeks of pregnancy) and up to 69% accuracy for preterm birth (births before 37 weeks of pregnancy). These models are based on the vaginal microbiome during pregnancy, which varies in composition and is key to a healthy pregnancy. The full results were published in Cell Reports Medicine.
Overcoming Data Restrictions
Machine learning models are strengthened by having robust data to learn from and analyze, but all the data needs to be in the same format. Vaginal microbiome data is generated by investigating various regions of a single gene, meaning the data between studies is not uniform.
Harmonizing microbiome data is a complex task, and previous attempts to study the microbiome generally only used single datasets with limited samples. To overcome this limitation, co-first author Jonathan Golob, MD, PhD, assistant professor of internal medicine at the University of Michigan, created an open-source tool called MaLiAmPi that brings microbiome data from disparate sources into a unified format so it can be used by machine learning models.
With this breakthrough tool, data from nine studies and over 1,200 pregnant people were combined into one comprehensive dataset for the public challenge, setting the stage for machine learning experts to make and submit reliable predictive models.
Moving Toward Clinical Application
Over 300 teams from around the world submitted machine learning models, which were tested against two previously unavailable datasets to determine their predictive power. Tomiko Oskotsky, MD, co-first author and senior research scientist in the Sirota Lab at UCSF, says having multiple, independently developed models with strong predictive powers strengthens the team’s confidence that the vaginal microbiome can be used in clinical applications to accurately identify pregnancies at risk for preterm labor.
Known as a DREAM Challenge, this crowd-sourcing approach required work from over 50 authors to define the prediction task, provide the necessary data, and evaluate all submitted models so that the global research community could be leveraged to create the best predictive models possible.
“Our team has now aggregated the best performing submissions into a single ensemble model, which we hope to test prospectively in a clinical trial with diverse populations. Once we’re able to reliably assess which pregnancies are at risk, we can better support those pregnancies and develop new interventions to protect the parent and child,” says Sirota.