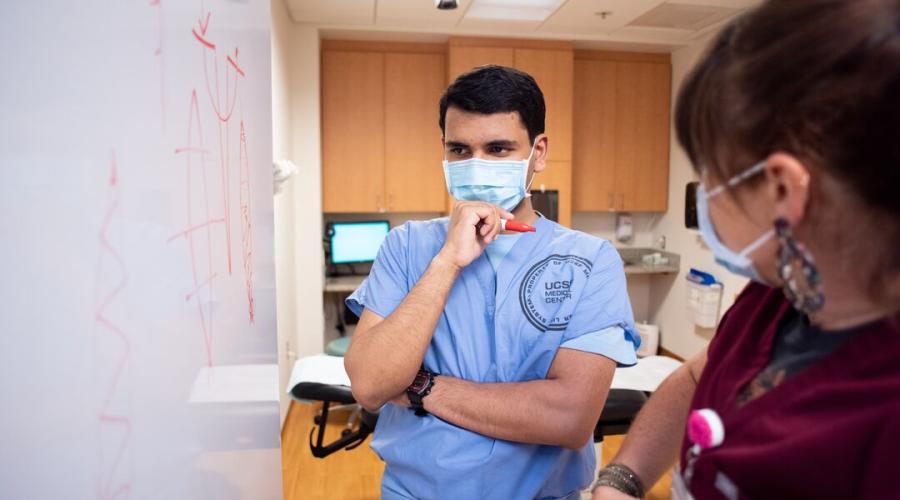
Study Reveals Discrepancies in How Medical Educators and Trainees Assess Competence
Medical trainees gain autonomy in caring for patients by earning their supervisors’ trust in their competence, but a recent study from UC San Francisco reveals a disconnect in how competence is assessed.
While trainees considered a wide range of factors when judging their own performance, including clinical skills and communicating well with patients, supervisors prioritized patient presentations where the trainee provides their assessment of the patient and plan for treatment.
“Even though both parties valued the clinical competencies of the trainee, the difference in which competencies they focused on shows the need for more collaborative approaches for determining a trainee’s trustworthiness and appropriate level of autonomy,” says Brian Gin, MD, PhD, an associate professor of pediatrics in the Division of Hospital Medicine and first author of the study.
Examining the Disconnect
The study, published in Advances in Health Science Education, used artificial intelligence to analyze over 24,000 written notes from feedback conversations following observed clinical tasks to identify themes and patterns in the language used by trainees and supervisors. The dataset also included numerical rankings of the amount of supervision the trainee needed during the clinical encounter, called entrustment ratings.
By comparing the language used in comments from trainees and supervisors, the study showed the supervisors tended to use more positive language than trainees when documenting feedback. Often, supervisors used the proverbial “feedback sandwich”:
"Good job doing a comprehensive history and physical examination on a patient with exacerbation of congestive heart failure. I was impressed with the level of detail and the thoroughness of the presentation. As we discussed, I would start to think about what information needs to be a part of an oral presentation, versus what important information can simply be recorded in your written note for reference. This will help to make presentations more concise and easier to follow. Great start!"
In contrast, a trainee’s summary of feedback in a similar scenario directly points to areas for them to improve on:
"Overall, ***'s presentation of their patient during our coach-led preceptorship was comprehensive. Some points to work on: 1) non-pertinent information in HPI [history of present illness] can be moved to ROS [review of systems] to streamline narrative and 2) organize medication list by diagnosis (will be helpful to listener and help them follow along)."
Despite using less positive language, trainees tended to give themselves higher entrustment ratings than their supervisors, indicating that they felt they had been trusted to act with more autonomy than a supervisor’s assessment of the situation.
Improving Practices
Gin, who studies the patient-supervisor-trainee dynamic, encourages training curricula that enable supervisors and trainees to find a shared understanding around entrustment.
“When trainees take an active role in determining their level of autonomy, the dynamic of mutual trust emerges. Curricula and assessment methods that emphasize this bidirectionality of trust are in development and beginning to show promising results,” says Gin.
Artificial Intelligence and Medical Education
Traditionally, qualitative and quantitative methods have been seen as distinct approaches: the former excels at capturing the nuance and context of human experience, and the latter harnesses statistics to detect patterns.
Here, artificial intelligence aided the researchers to analyze both the language and the numerical rankings documented by trainees and supervisors, blurring the lines between qualitative and quantitative traditions to generate comprehensive insights from over 24,000 written entries that would be difficult to analyze manually and with consistency.
Sandrin van Schaik, MD, PhD, Vice Chair for Education for the UCSF Department of Pediatrics, says “this study highlights how artificial intelligence can help educational scholars by training large language models to detect bias towards (or coming from) particular groups. These insights can serve to inform interventions to mitigate such bias, and then measure the impact of those interventions.”